New Professor for Teaching and Learning in the Digital World at CAU and IPN
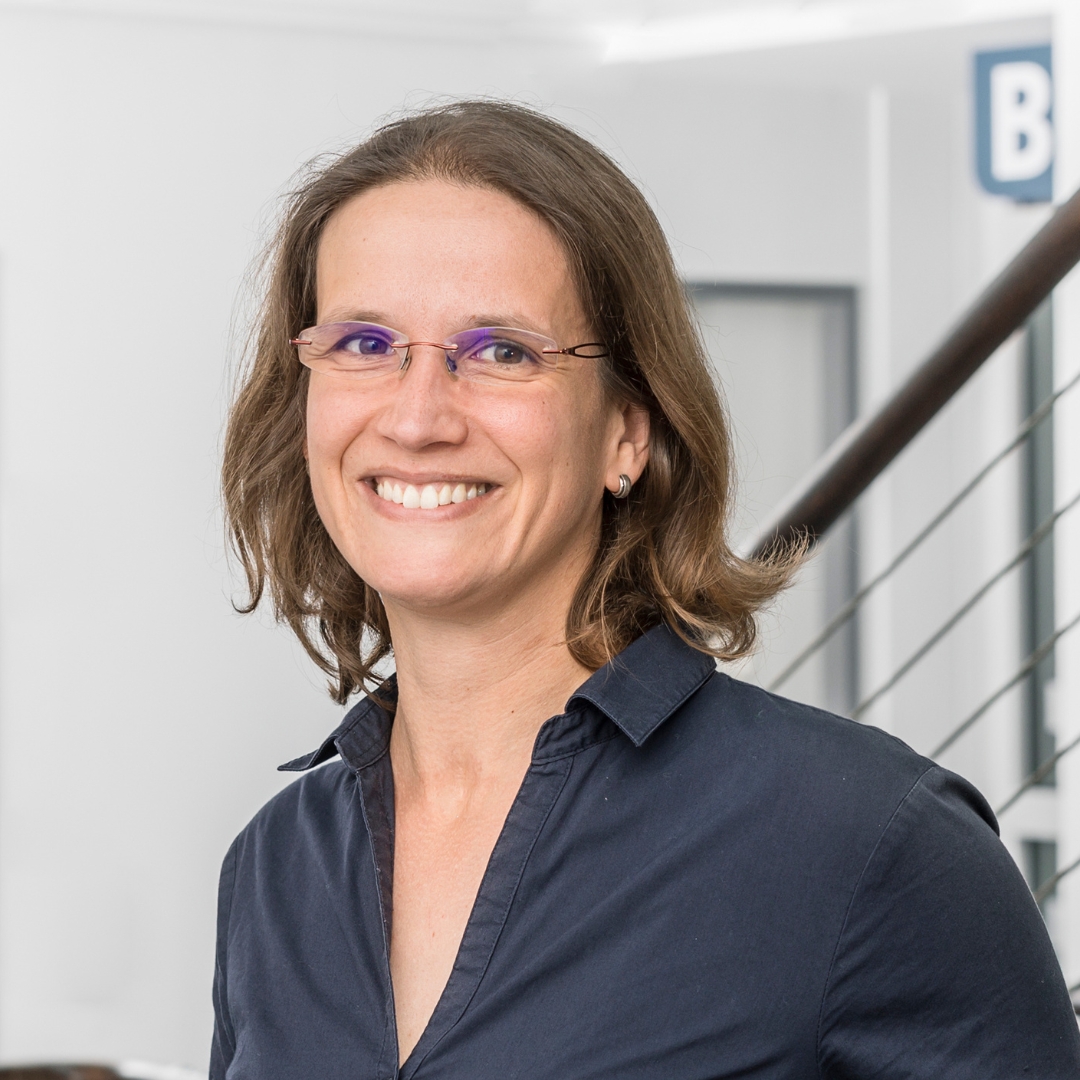
Kiel University (CAU) and the Leibniz-Institute for Science and Mathematics Education (IPN) have jointly appointed Prof. Dr. Andrea Horbach to a professorship of Teaching and Learning in the Digital World, with a focal emphasis on machine learning and learning analytics. Prof. Horbach, whose appointment is effective September 2024 and is at W3 level in the German system, will accordingly be contributing her expertise both to the Institute of Educational Sciences (IPL) at CAU and to the IPN’s Department of Educational Measurement and Data Science.
A computational linguist, Prof. Horbach completed degree and doctoral studies at Saarland University in Saarbrücken. One of her key research interests in her career to date has been the field of educational natural language processing (EduNLP), whose purpose is to explore ways in which language technologies can support teachers and learners. After leaving Saarbrücken, Prof. Horbach took up a postdoc position in the Language Technology Lab of the University of Duisburg-Essen; in 2021, she moved to CATALPA (Center of Advanced Technology for Assisted Learning and Predictive Analytics) at Hagen’s distance-learning university FernUniversität, where she took charge of the EduNLP Junior Research Group. There followed a junior professorship in Digital Humanities at the University of Hildesheim, which she commenced in the summer semester of 2023.
‘As a computational linguist, I’ve always been fascinated by the way computers can generate, analyze and understand language despite it being so complex and full of ambiguities. In terms of education, language is both a skill that learners need to acquire and the most natural channel of human communication.’
Prof. Horbach will now be contributing her expertise in machine learning and learning analytics to research and teaching at Kiel’s CAU and IPN. Much of her work centers on the use of NLP and AI in education, which includes automatically generating and marking work for learners and giving feedback via AI systems. From her doctoral dissertation onward, she has had a special interest in the automatic assessment of learners’ textual answers and its impact on teaching and learning processes. One of the aims of her work is to provide teachers with wording suggestions for assessment that they feel are appropriate and usable, thus reducing their workload, and simultaneously to improve the quality of the feedback learners receive. Prof. Horbach is also exploring the potential of harnessing data from writing processes so that students can receive supportive feedback at an early stage of their learning.
‘In my view, research questions of this type need an interdisciplinary approach – and Kiel offers an ideal setting for this work.’
Prof. Horbach is keen to emphasize the crucial significance of interdisciplinary research in the study of these fields. A major influence on her view of Kiel as an optimal location for advancing this research is the opportunity it provides for collaboration with IPN and IPL staff with whom she worked previously on projects including TRACE and KISS-Pro.
We caught up with Prof. Horbach to find out more about the foci of her research, and asked her about her plans for her work in Kiel and about possibilities for new projects going forward.
IPN: Congratulations on your appointment and welcome to Kiel! Your new job revolves around ‘teaching and learning in the digital world’, specifically around machine learning and learning analytics. What is it about this particular combination that you find most exciting?
Prof. Horbach: As a computational linguist and someone who works with language technologies, I dedicate myself to discovering and developing ways of automatically analyzing and generating human language. This is a field with massive potential in the educational arena – after all, teaching is impossible without language, and that’s as much the case for science subjects as for any other. In terms of language technologies, there are lots of possible ways of supporting [the teaching and learning process], such as generating questions or reading tasks for learners at appropriate levels of difficulty, automatic assessment of learners’ writing, and automated feedback.
Machine learning methods enable computers to use large volumes of data to learn algorithms that can transfer the knowledge in those data to new data points. This means – to give an example – that when we’ve learned how to assess an argumentative essay, we can apply that classifier to essay after essay without a teacher having to manually assess them. This can help us support learners as well as saving teachers work on time-consuming tasks such as marking homework.
IPN: In your career to date, you’ve worked closely on educational natural language processing (EduNLP). What particularly promising developments can you currently see in this field, and to what extent are they making themselves felt in your research?
Prof. Horbach: There are a few trends right now in the NLP world that are especially significant for EduNLP. In the last few years, the previous exclusive focus on performance has begun to broaden, and the explainability of algorithms is now gaining in importance. Education is one sector that absolutely depends on explainable algorithms that teachers and learners can trust, whether we use explainability to increase users’ confidence in the machine or whether we want to deploy explainability methods specifically for feedback purposes. Better explainability has the additional advantage of enabling us to recognize potential biases in algorithms.
Another key development is that research into NLP is no longer focusing only on English-language data; there’s a new appreciation for linguistic diversity, and we’re now in a situation where publications on German data, for example, are welcomed internationally. Of course, machine learning needs large datasets, and it’s great that increasing volumes of data are available in a wide range of languages. I see a lot of potential in looking beyond completed pieces of writing by learners and starting to make use of data from the process, such as keylogs or eye-tracking - ideally, this will help us, for instance, to give feedback during the actual writing process, rather than waiting until the essay is finished.
It goes almost without saying that the wide availability of generative large language models has opened up a lot of possibilities for new applications in the education sector and that it’ll have a long-term impact on teaching and learning going forward. In this context, researchers working on NLP are asking questions like what LLMs can do and what they can’t do (yet), and how we can combine more traditional methods with LLMs in a helpful way.
IPN: What do you see as the opportunities and challenges of incorporating machine learning and learning analytics into the day-to-day business of teaching?
Prof. Horbach: The major opportunity that machine learning and learning analytics present in terms of teachers’ work consists in the anytime, anyplace availability of immediate feedback to learners, meaning they are no longer dependent on teachers – who are finite resources - for getting feedback on their work. In the ideal case, the use of AI in education would provide every learner with their own automated tutor that knows their strengths and skills and can give them personalized support.
This, of course, gives rise to a whole bunch of challenges. What does optimal feedback look like, and how can we generate it automatically, ideally without having to train it on overly large volumes of data? Are the algorithms used to assess learners actually fair? What biases are built into feedback algorithms and how do they differ from the biases that a human teacher has? How do we handle the routine use of AI tools by learners for things such as writing tasks?
Then there are very practical issues, such as how well equipped a school is with tech and the extent to which the teaching staff are happy to use AI.
IPN: What work, specifically, are you planning to do at CAU and IPN? Do you have any projects or initiatives that you’re especially excited about?
Prof. Horbach: I’m currently busy getting my new team set up – I’m incredibly lucky to be able to still work with some highly motivated doctoral students based at the University of Hildesheim and at CATALPA in Hagen and to continue some existing collaborative projects. One of the core areas of work in this context is automated assessment of argumentative writing; it identifies a range of argumentative units in learners’ essays written in school, and shows where the arguments are in the writing and where the introduction and conclusion are. I’m working with one of my doctoral students in Hagen, Yuning Ding, to find ways of transferring these algorithms to new contexts; one thing we’re looking at is transfer to new tasks or questions, but also transfer between different learning scenarios - for example, how do essays written in German classes in Germany differ from those written in American high schools, and what are the implications for their automated assessment? We’re working closely with the IPN’s DARIUS junior research group on this.
Another core area of our work is automated assessment of short answers [to questions], which is a format that major student assessment studies use. I’m working with Marie Bexte, a doctoral student at CATALPA, and other colleagues in Hagen and at the DIPF [Leibniz Institute for Research and Information in Education] in Frankfurt, focusing on cross-lingual approaches to this area, where, for instance, the training data for an algorithm are in a language that’s different from the writing we want to use the algorithm on.
A third focal field relates to linguistic phenomena that affect the difficulty of text written for learners or that are indicative of a learner’s level of language development. One example of my work in this area is my collaboration with the TestDaF-Institut [an organization providing testing for proficiency in German as a foreign language] on comparing linguistic characteristics of text for training learners’ reading skills, that either a human or generative AI has written. Also I’m working with Anna Hülsing, one of my doctoral students from Hildesheim, to study the automated recognition of metaphors in essays, which is a characteristic of skilled language use.
Alongside this, various ideas for other projects are in progress, on topics such as explainable support for teachers in the assessment process, work with process data, and feedback on writing in the foreign language classroom. My dual base with this professorship, at the IPN and the IPL, puts me in a great position to initiate new collaborative endeavors.
IPN: What does it mean to you personally to have accepted a professorship in an area that’s growing so fast and dynamically?
Prof. Horbach: I’m really pleased to have a long-term opportunity here in Kiel to bring together the fields I’m passionate about - language, computing and education - and to set up my own research program. Kiel provides me with the perfect setting for all this.
Of course, there’s also a lot going on internationally in the area of digital education. In the last two years, as co-organizer of the Building Educational Applications Workshop run by the ACL (Association for Computational Linguistics), I’ve seen a huge boom in submissions of papers from a really wide variety of countries, which I’m delighted about, because the more interest there is in the field, the more exciting possibilities for potential collaborative projects open up.